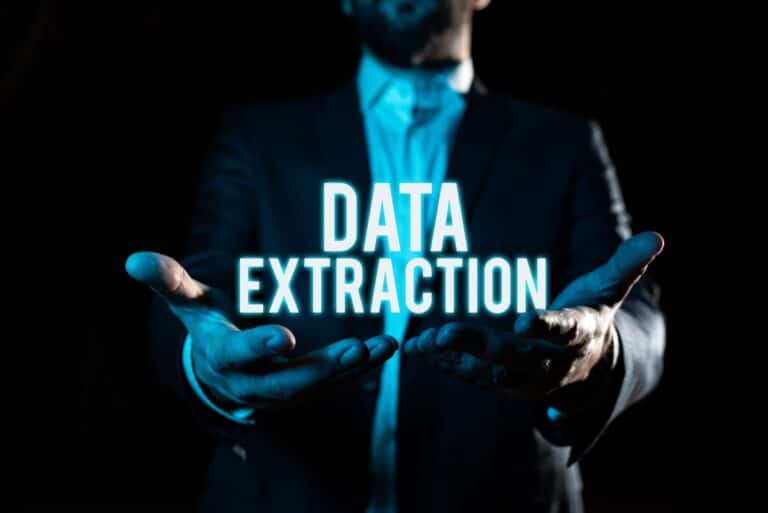
EMR data extraction can be a complex task. Apart from the wide range of options available to you, your EMR contains a mixture of structured and unstructured data.
While most EMR data extraction activities require pulling data from normalized, structured data elements, there’s a significant volume of unstructured data that must not be ignored. The first step in obtaining existing legacy patient data, proper EMR data extraction, means exporting as much data as possible in a usable format in order to seamlessly migrate to the new system.
- What is EHR Data Extraction
- What is structured and unstructured healthcare data
- How is legacy EMR data extracted?
- Challenges in EHR/EMR Data Extraction
What is EHR Data Extraction
EHR data extraction is one of three essential alternatives to move all data from a legacy EHR system to a new system and is done through a process known as ETL. During this process, patient data is EXTRACTED from the legacy system, TRANSFORMED to align with the map created for the new system, and LOADED into the new system.
What is structured and unstructured healthcare data?
Healthcare organizations rely on the power of data to improve operational efficiency and gain valuable insight into patient health and treatment. However, of the volume of data that is available, only 10% – 20% of it is usable. As a result, 80% – 90% of healthcare unstructured data is not leveraged because it is too challenging to interpret.
Structured data is often managed and searched through an online database or through an online database management system (RDBMS). This includes integers, decimals, dates, time, strings, and Booleans. These information varieties are unit simply organized in rows and columns and may be queried by victimization programming languages like SQL to come back with relevant search data. They will be mechanically combined and processed as a result of their easy boundaries and are made and kept in a standardized format.
Examples of Structured Data in Healthcare
In care, structured information is preponderantly wont to record patient data in electronic health records (EHR). Here area unit some examples:
- Medical take a look at results are often recorded within the variety of a numeric or Boolean worth.
- Physical measures like height, weight, pressure level, blood type, and stage of the sickness are often recorded numerically.
- Dropdown menus are often used for storing demographic data.
- A radio button can be used to denote the patient’s gender, marital status, and other binary values.
In every example, what makes the values structured is the ease with which they will be parsed by computers and queried by humans. In an endeavor to create clinical information, even a lot of uniform and accessible comes just like the Structured Data Capture (SDC) Profile has provided associate infrastructure for capturing, exchanging, and utilizing EHR information to enhance clinical analysis, enhance adverse event coverage, and optimize public health coverage.
Unstructured data, on the other hand, isn’t computer code and thus needs extended pre-processing in preparation to be used with analysis tools.
Unstructured Data Examples in Healthcare
Unstructured health data remains one of the most precious and untapped resources in the healthcare ecosystem. While determined medical professionals can certainly make use of this data, the process is time-consuming and fails to produce an overall, cohesive picture. Here are some examples of unstructured data:
- Medical images such as PET, CAT, and MRI scans, X-rays, and ultrasounds.
- Audio recordings from therapy sessions.
- Text files of varying lengths, such as medical notes and evaluations.
How is legacy EMR data extracted?
Legacy systems in healthcare such as Electronic Health Records (EHRs) and Electronic Medical Records (EMRs) software companies want to convince you that you must keep paying subscription fees to maintain access to your facility’s legacy data. The truth is the information is yours. Learn how to easily extract legacy data and transfer it into an easily accessible format.
Legacy systems in healthcare are dangerous. Legacy systems in healthcare such as Electronic Health Records (EHRs) and Electronic Medical Records (EMRs) software companies want to convince you that you must keep paying subscription fees to maintain access to your facility’s legacy data. The truth is the information is yours. Contact us to learn how to easily extract legacy data and transfer it into an easily accessible format.
- System integrity – Legacy systems still require annual subscription fees, but archaic platforms are less likely to be continuously upgraded. The original software developers may not be at the company and even if they are, they are working on the latest version rather than the old system. This means that the integrity of the entire system and its data is at risk from cyberattacks and hardware failures.
- Access to information – You know passwords can be lost, new staff aren’t trained on old systems, or previous hardware is housed elsewhere. These situations make auditing and legal requests for information an administrative nightmare. The legislation mandates access to information for up to 10 years, so you need quick access to archived information.
- Patient care – Technically this is also access to information, but the only reason medical facilities exist is to help patients. Patients sitting in examination rooms suffer (or complain!) when information is restricted to those who have passwords and access to the system
Challenges in EHR / EMR Data Extraction
Data access and privacy – Due to the high sensitivity of data collected by EHR systems, access to clinical data such as electronic medical records is highly restricted. Ethical clearance is required, as well as good communication with healthcare providers. The reuse of such data for research and quality improvement work is subject to multiple levels (GDPR (2016), HIPAA (2003), and national and institutional policy). At the same time, there are forces demanding that the valuable information available within the healthcare system be used not only for research but also for improving healthcare.
Data quality is a major issue and we need ways to improve data integrity, compliance, and validity.
Statistical analysis – Data collected from EHRs may have higher error rates than data collected using proprietary systems and subjected to “cleansing” and validation. Missing data, including those resulting from cluster-wide failures, pose certain problems for PCT. Preliminary data collection and evaluation provide an indication of whether a proposed study is viable given data availability and quality.